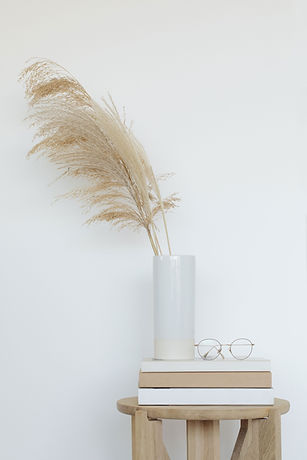
Adversarial-Enhanced Hybrid Graph Network for User Identity Linkage
Abstract
Recently, people tend to engage themselves in multiple social media platforms simultaneously to enjoy various services. As a pivotal prerequisite of many cross-platform tasks, user identity linkage across different social platforms has gained tremendous research attention. Existing efforts mainly focus on investigating either the textual posts, check-in posts, social connections, or the combinations of them, but overlooking the visual cues in the user characterization. In light of this, we aim to comprehensively fulfil the user identity linkage task by additionally incorporating the visual cues of the user’s posts, apart from the textual post, check-in posts, and social connections. Accordingly, we propose a novel adversarial-enhanced hybrid graph network (AHG-Net), which consists of three key components: user representation extraction, hybrid user representation learning and adversarial learning. AHGNet first employs advanced deep learning techniques to extract the user’s intermediate representations from his/her heterogeneous multi-modal posts as well as social connections. Then AHGNet unifies the intra-user representation learning and inter-user representation propagation with a hybrid graph network. Finally, beyond existing studies, we adopt adversarial learning by treating the user representation learning as a generator, and additionally introduce a semantic discriminator to distinguish the platform source of each user representation, which essentially promotes the learned user presentations of the same identity to be similar. Towards evaluation, we create a multi-modal user identity linkage dataset by augmenting an existing dataset with 62, 021 images collected from Twitter and Foursquare. Extensive experiments on this dataset validate the superiority of the proposed network. As a byproduct, we have released the dataset, codes, and parameters to facilitate the research community.
Framework
Illustration of the proposed network for user identity linkage.

Copyright
Permission to make digital or hard copies of all or part of this work for personal or classroom use is granted without fee provided that copies are not made or distributed for profit or commercial advantage and that copies bear this notice and the full citation on the first page. Copyrights for components of this work owned by others than ACM must be honored. Abstracting with credit is permitted. To copy otherwise, or republish, to post on servers or to redistribute to lists, requires prior specific permission and/or a fee. Request permissions from permissions@acm.org.